[ad_1]
Much less is Extra? Lowering Biases and Overfitting in Machine Studying Return Predictions
Machine studying fashions have been efficiently employed to cross-sectionally predict inventory returns utilizing lagged inventory traits as inputs. The analyzed paper challenges the standard knowledge that extra coaching knowledge results in superior machine studying fashions for inventory return predictions. As a substitute, the analysis demonstrates that coaching market capitalization group-specific machine studying fashions can yield superior outcomes for stock-level return predictions and long-short portfolios.
The creator evaluates the efficiency of three fashions educated on non-overlapping teams of shares based mostly on their market capitalization (massive, mid, and small-cap) and finds vital enhancements in return predictions and portfolio efficiency. These findings have implications for each teachers and practitioners within the area of finance, emphasizing the necessity for considerate mannequin design and the potential advantages of group-specific modeling. The examine additionally conducts simulations to evaluate the generalizability of those outcomes past the U.S. market, additional contributing to the literature on machine studying in asset pricing.
Desk 3 offers an insightful comparability of long-short portfolios fashioned by sorting shares based mostly on their extra return predictions. These portfolios are held for one month and earn both value-weighted (VW) or equal-weighted (EW) returns. The desk presents varied key efficiency statistics for 9 predictive fashions and two ensembles, evaluating fashions educated on the total cross-section of shares (Full), group-specific fashions based mostly on market capitalization (Dimension), and an ensemble of each (Ensemble). The outcomes from Desk 3 constantly present that coaching on group-specific fashions (Dimension) results in stronger portfolio traits. For the ensemble of all fashions, the annualized portfolio return will increase considerably, from 20.0% for the Full mannequin to 31.8% for the Dimension mannequin. Whereas this enhance is accompanied by larger portfolio volatility, the Sharpe ratio additionally will increase, indicating that the extra threat is compensated by the improved return. These findings underscore the effectiveness of group-specific modeling in enhancing portfolio efficiency, and this influence just isn’t solely attributed to elevated portfolio buying and selling.
With no normal framework for mannequin comparability, the analysis explores the complexity of machine studying modeling decisions in asset pricing. By coaching group-specific machine studying fashions, the examine demonstrates their superior predictive and portfolio efficiency when in comparison with fashions educated on the total dataset.
We additionally suggest reviewing Determine 3, which reveals the relative significance of options when coaching in measurement classes. The determine offers a pleasant overview of what are vital inputs within the ML mannequin and is an effective addition to Displays 4 and 5 from our earlier weblog that analyzed reducing returns of machine studying methods.
Writer: Clint Howard
Title: Much less is Extra? Lowering Biases and Overfitting in Machine Studying Return Predictions
Hyperlink: https://papers.ssrn.com/sol3/papers.cfm?abstract_id=4497739
Summary:
Machine studying has grow to be more and more widespread in asset pricing analysis. Nonetheless, widespread modeling decisions can result in biases and overfitting. I present that group-specific machine studying fashions outperform fashions educated on a broader cross-section of shares, difficult the widespread perception that extra knowledge results in higher machine studying fashions. The superior efficiency of group-specific fashions may be attributed to a scarcity of regularization of the goal inventory returns. Coaching on uncooked inventory returns produces fashions that overfit to predicting the returns of smaller shares, decreasing the efficiency of value-weighted buying and selling methods. Easy changes to the goal, comparable to eradicating the cross-sectional measurement–group median, produce related financial beneficial properties because the group–particular fashions with out the added computational value. These findings emphasize the cautious steerage required when designing and making use of machine studying fashions for cross-sectional return prediction.
As at all times, we current a number of attention-grabbing figures and tables:
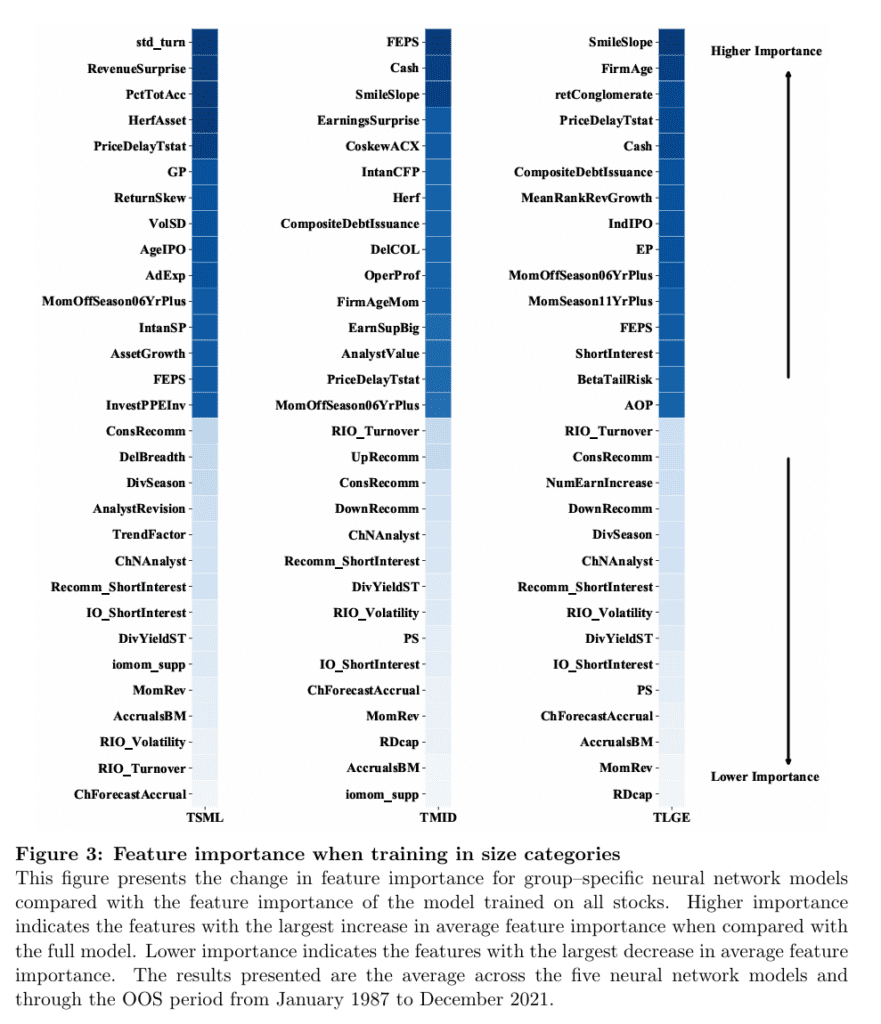
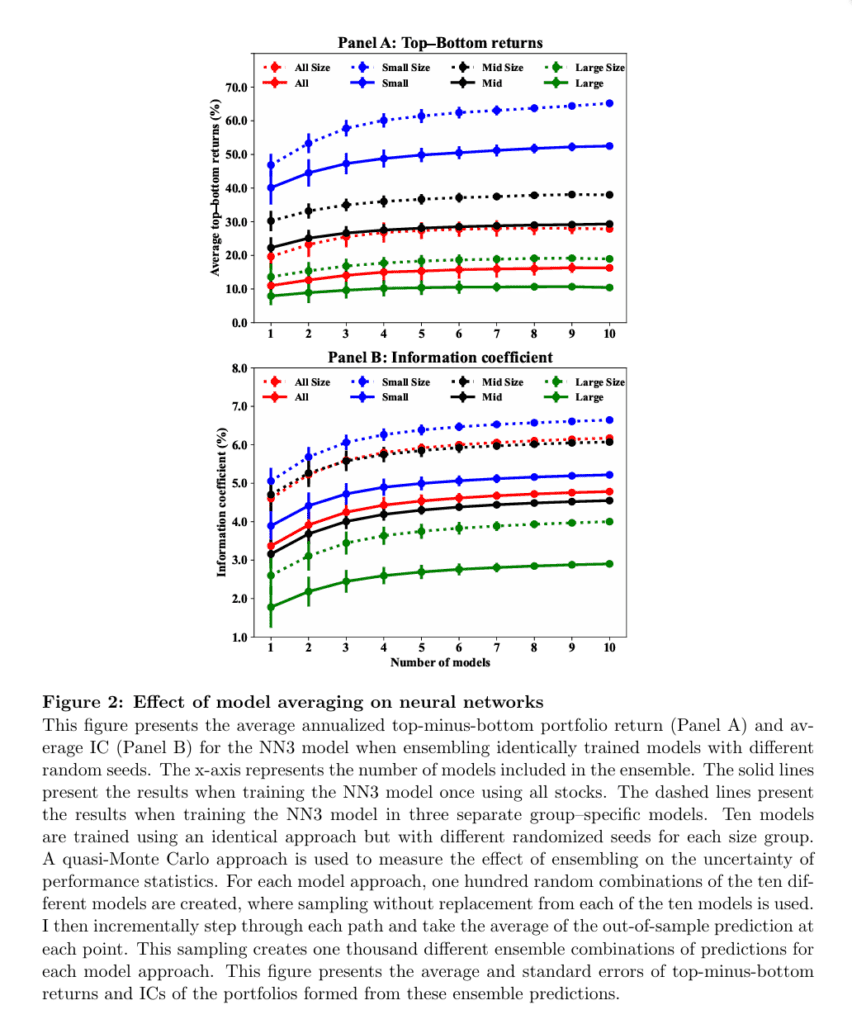
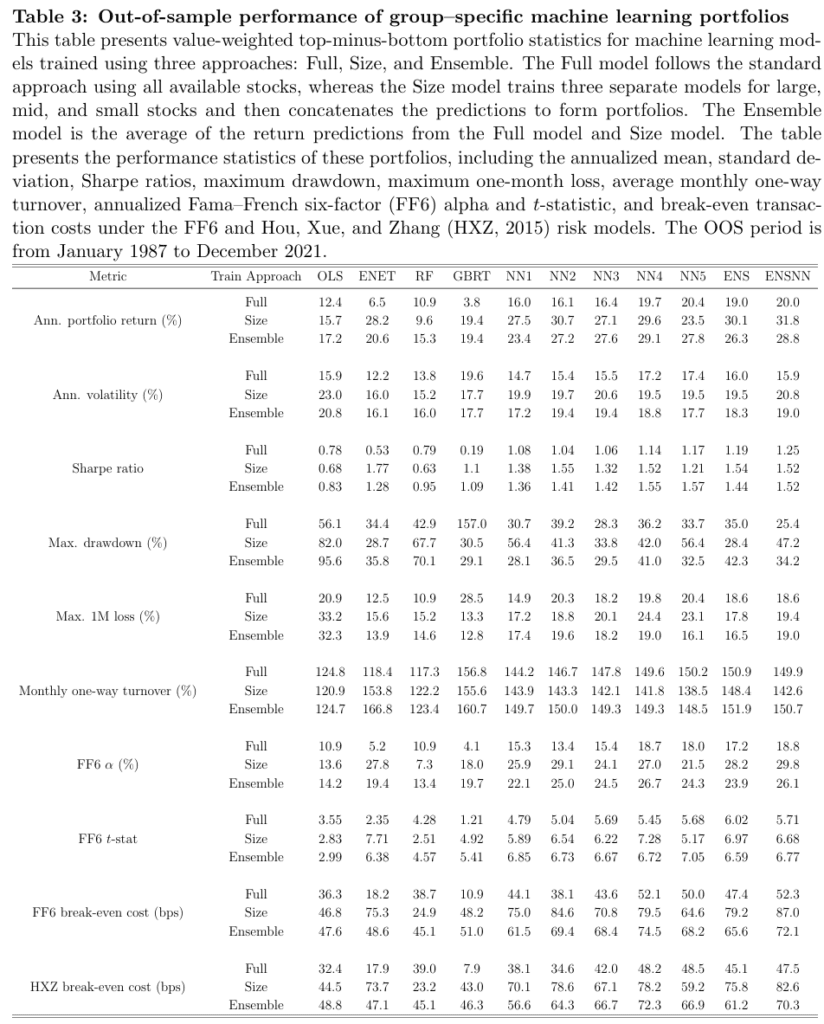
Notable quotations from the educational analysis paper:
“In designing and estimating machine studying fashions, I observe the overall empirical setupof Guet al. (2020). I exploit Chen and Zimmermanns (2022) Open Supply Asset Pricing (OSAP)database for month-to-month stock-level traits, and I don’t embrace any macroeconomiccovariates within the examine. As well as, I deal with group-specific machine studying fashions,the place I individually practice machine studying fashions for diferent size-groups of shares.
The outperformance of group-specific machine studying fashions poses a problem to thecommonly held perception that extra coaching knowledge result in superior efficiency of machinelearning fashions. To evaluate whether or not this anomaly is primarily a function of the U.S. CRSPdata setting or a generalized outcome for machine studying fashions, I conduct a simulation studyusing group-specific dependencies between simulated enter options (inventory traits)and outputs (inventory returns) and fluctuate the degrees of volatility and predictive efficacy withinthese teams. I observe the fundamental DGP setup from Gu et al. (2020) with augmentationsthat simulate a conditional dependence between covariates. The Appendix incorporates the fulldetails on the simulation strategy.
Finally, we have an interest within the sensible utilization of machine studying fashions for asset pricing and portfolio administration functions. The habits of machine studying modelsusing simulated issue DGPs offers insights into the underlying mechanics however is proscribed in sensible relevance. By way of the simulation train, I discovered that neural community modelscan over t teams of property inside the coaching dataset. Utilizing this perception, I now conductempirical experiments to research how machine studying design choices have an effect on mannequin efficiency and which design decisions can scale back this group-specific overftting. Specifically,I deal with three essential areas of mannequin design choices: options, structure, and goal.I make stylized decisions inside every class and analyze their influence on stock-level returnpredictions and portfolio efficiency. I don’t purpose to cowl each doable modeling determination however quite to discover the widespread consultant decisions noticed in literature andadditional circumstances associated to the group-specific mannequin outcomes. I completely deal with the NN3model, given the upper propensity for overfitting of neural community architectures have foroverfitting in contrast with tree-based fashions.
Finance literature has solely simply begun to discover the applying of machine studying fashions for predicting cross-sectional inventory returns. There is no such thing as a normal modeling body work for evaluating outcomes throughout completely different research. The excessive dimensionality of decisions related to machine studying modeling in asset pricing leads to a excessive degree of complexity in attributing efficiency beneficial properties associated to adjustments to machine studying modeling approaches. This examine contributes to the eld by coaching group-specific machine studying fashions and demonstrating superior predictive and portfolio efficiency in contrast with a mannequin educated on the total dataset. By investigating varied machine studying design decisions, I reveal {that a} lack of regularization of the goal variable primarily drives the outperformance of group-specific machine studying fashions. By implementing goal variable regularization, the efficiency beneficial properties related to group-specific machine studying fashions may be achieved at decrease computational complexity.”
Are you on the lookout for extra methods to examine? Join our publication or go to our Weblog or Screener.
Do you wish to study extra about Quantpedia Premium service? Examine how Quantpedia works, our mission and Premium pricing supply.
Do you wish to study extra about Quantpedia Professional service? Examine its description, watch movies, evaluate reporting capabilities and go to our pricing supply.
Are you on the lookout for historic knowledge or backtesting platforms? Examine our record of Algo Buying and selling Reductions.
Or observe us on:
Fb Group, Fb Web page, Twitter, Linkedin, Medium or Youtube
Share onLinkedInTwitterFacebookConfer with a buddy
[ad_2]
Source link