[ad_1]
Design Decisions in ML and the Cross-Part of Inventory Returns
Current developments in machine studying have considerably enhanced the predictive accuracy of inventory returns, leveraging complicated algorithms to investigate huge datasets and determine patterns that conventional fashions typically miss. The most recent empirical research by Minghui Chen, Matthias X. Hanauer, and Tobias Kalsbach reveals that design selections in machine studying fashions, resembling characteristic choice and hyperparameter tuning, are essential to enhancing portfolio efficiency. Non-standard errors in machine studying predictions can result in substantial variations in portfolio returns, highlighting the significance of strong mannequin analysis methods. Integrating machine studying methods into portfolio administration has proven promising leads to optimizing inventory returns and general portfolio efficiency. Ongoing analysis focuses on refining these fashions for higher monetary outcomes.
Present analysis reveals substantial variations in key design choices, together with algorithm choice, goal variables, characteristic therapies, and coaching processes. This lack of consensus leads to important consequence variations and hinders comparability and replicability. To handle these challenges, the authors current a scientific framework for evaluating design selections in machine studying for return prediction. They analyze 1,056 fashions derived from numerous combos of analysis design selections. Their findings reveal that design selections considerably affect return predictions. The non-standard error from unsuitable selections is 1.59 occasions increased than the usual error.
Key findings embrace:
ML returns fluctuate considerably throughout design selections (see Determine 2 under).
Non-standard errors arising from design selections exceed customary errors by 59%.
Non-linear fashions are likely to outperform linear fashions just for particular design selections.
The authors present sensible suggestions within the type of actionable steering for ML mannequin design.
The research identifies essentially the most influential design selections affecting portfolio returns. These embrace post-publication remedy, coaching window, goal transformation, algorithm, and goal variable. Excluding unpublished options in mannequin coaching decreases month-to-month portfolio returns by 0.52%. An increasing coaching window yields a 0.20% increased month-to-month return than a rolling window.
Moreover, fashions with steady targets and forecast combos carry out higher, highlighting the significance of those design selections. The authors present steering on choosing acceptable choices based mostly on financial results. They advocate utilizing irregular returns relative to the market because the goal variable to realize increased portfolio returns. Non-linear fashions outperform linear OLS fashions below particular situations, resembling steady goal returns or increasing coaching home windows. The research emphasizes the necessity for cautious consideration and rational justification of analysis design selections in machine studying.
Authors: Minghui Chen, Matthias X. Hanauer, and Tobias Kalsbach
Title: Design selections, machine studying, and the cross-section of inventory returns
Hyperlink: https://papers.ssrn.com/sol3/papers.cfm?abstract_id=5031755
Summary:
We match over one thousand machine studying fashions for predicting inventory returns, systematically various design selections throughout algorithm, goal variable, characteristic choice, and coaching methodology. Our findings reveal that the non-standard error in portfolio returns arising from these design selections exceeds the usual error by 59%. Moreover, we observe a considerable variation in mannequin efficiency, with month-to-month imply top-minus-bottom returns starting from 0.13% to 1.98%. These findings underscore the essential affect of design selections on machine studying predictions, and we provide suggestions for mannequin design. Lastly, we determine the situations below which non-linear fashions outperform linear fashions.
As at all times, we current a number of bewitching figures and tables:
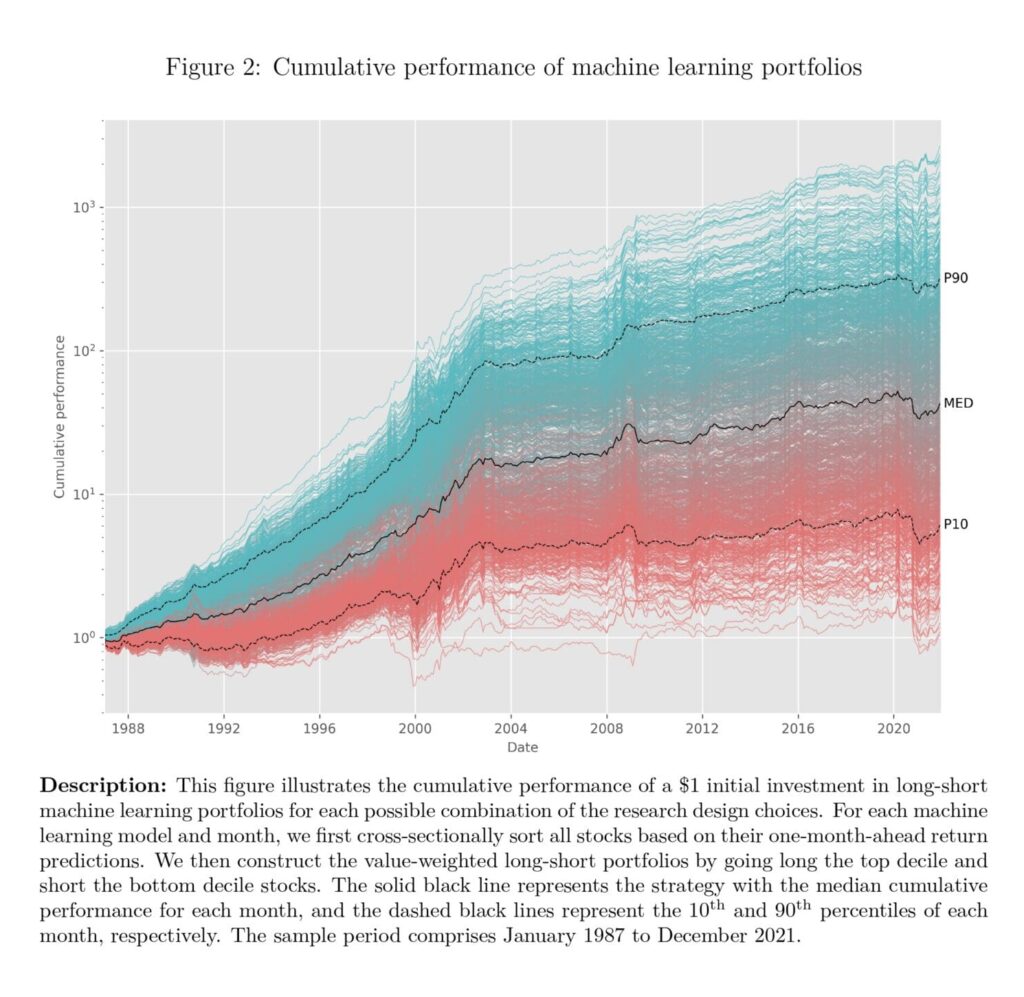
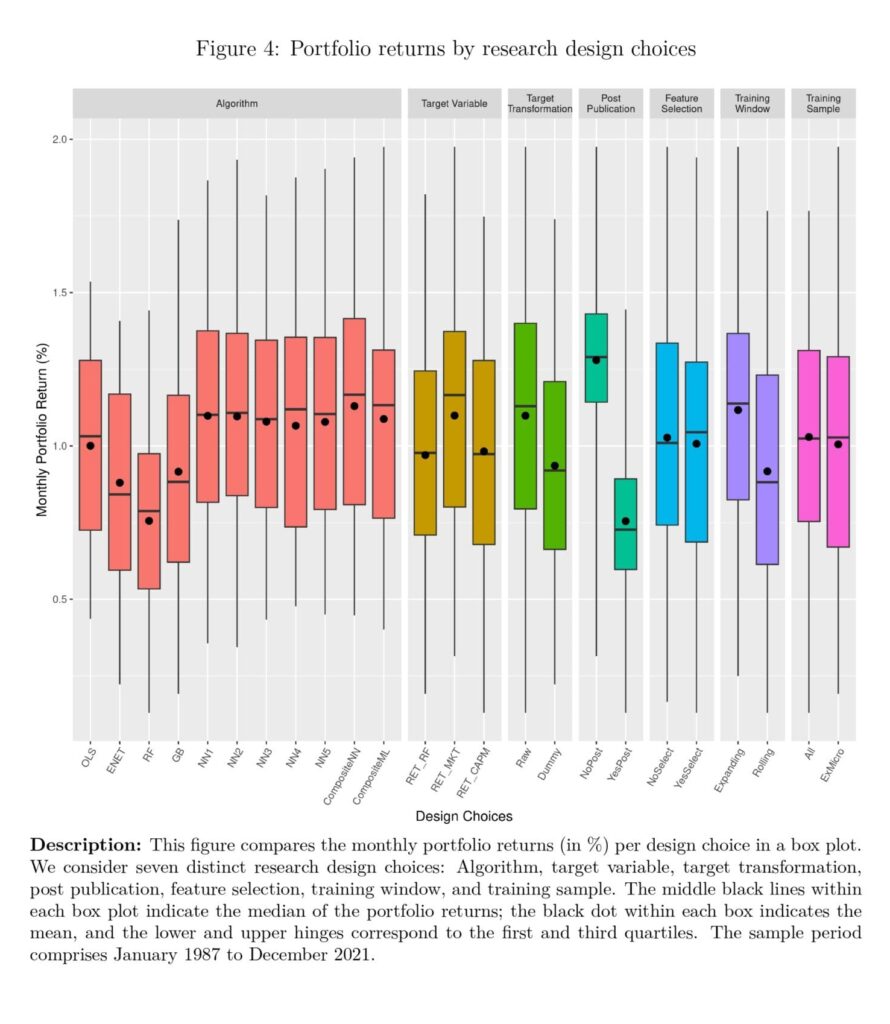
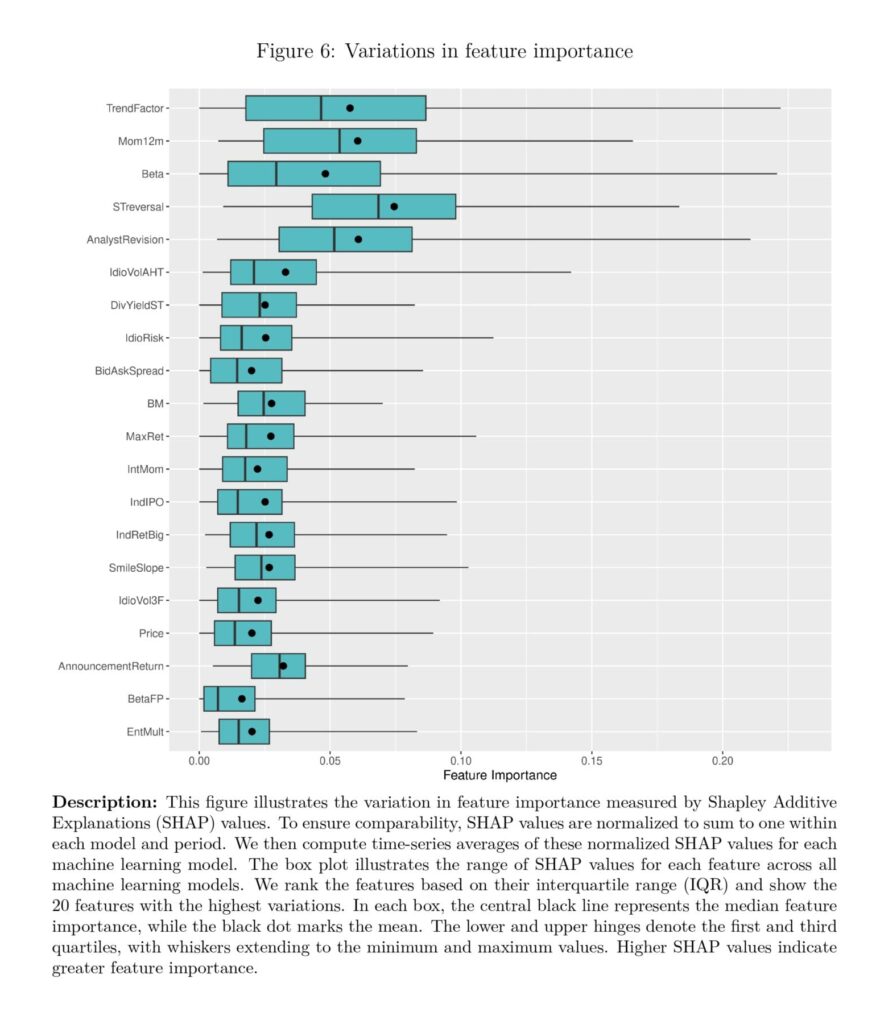
Notable quotations from the tutorial analysis paper:
“The primary findings of our research might be summarized as follows: First, we doc substantial variation in top-minus-bottom decile returns throughout totally different machine studying fashions. For instance, month-to-month imply returns vary from 0.13% to 1.98%, with corresponding annualized Sharpe ratios starting from 0.08 to 1.82.Second, we discover that the variation in returns attributable to these design selections, i.e., the non-standard error, is roughly 1.59 occasions increased than the usual error from the statistical bootstrapping course of.
[. . .] we contribute to research that present pointers for finance analysis. As an example, Ince and Porter (2006) supply pointers for dealing with worldwide inventory market knowledge, Harvey et al. (2016) suggest the next hurdle for testing the importance of potential elements, and Hou et al. (2020) advocate strategies for mitigating the affect of small shares in portfolio types. By providing steering on design selections for machine learning- based mostly inventory return predictions, we assist cut back uncertainties in mannequin design and improve the interpretability of prediction outcomes.
[. . .] research has necessary implications for machine studying analysis in finance. A deeper understanding of the essential design selections is crucial for optimizing machine studying fashions, thereby enhancing their reliability and effectiveness in predicting inventory returns. By addressing variations in analysis settings, our work helps researchers demon- strate the robustness of their findings and cut back non-standard errors in future research. This, in flip, permits for extra correct and nuanced interpretations of outcomes.
When predicting inventory returns utilizing machine studying algorithms, researchers and prac- titioners face a variety of necessary methodological selections. We determine such variations in design selections in a number of revealed machine-learning research, all of which predict the cross-section of inventory returns. Extra particularly, these research embrace Gu et al. (2020), Freyberger et al. (2020), Avramov et al. (2023), and Howard (2024) for U.S. market, Rasekhschaffe and Jones (2019) and Tobek and Hronec (2021) for international developed mar- kets, Hanauer and Kalsbach (2023) for rising markets, and Leippold et al. (2022) for the Chinese language market. In complete, we determine variations in seven frequent analysis design selections throughout these research, and we categorize them into 4 most important varieties relating to the algorithm, goal, characteristic, and coaching course of. Desk 1 summarizes the particular design selections of those research.
Subsequent, we examine the efficiency dispersion of the totally different machine-learning strate- gies ensuing from totally different design selections. Determine 2 reveals the cumulative efficiency of the 1,056 long-short portfolios. Every line represents the efficiency of 1 particular set of analysis design selections.The determine reveals that the variation in design selections results in a considerable variation in returns. A hypothetical $1 funding in 1987 results in a ultimate wealth starting from $0.94 (annual compounded return of -0.17%) to $2,652 (annual compounded return of 24.48%) in 2021. The most effective mannequin is related to design selections of Algorithm (ENS ML), Goal (RET-MKT, RAW), Function (No Put up Publication, No Function Choice), and Coaching (Increasing Window, ExMicro Coaching Pattern). Then again, the worst-performing mannequin is related to the design selections of Algorithm (RF), Goal (RET-CAPM, RAW), Function (Sure Put up Publication, Sure Function Choice), and Coaching (Rolling Window, All Coaching Pattern). The small print of the top- and bottom-performing fashions are documented in Appendix Desk B.2. Aside from that, we additionally observe that every one the machine studying fashions carry out worse in recent times, significantly after 2004, which aligns with the findings of Blitz et al. (2023).
Determine 4 reveals the portfolio returns in a field plot with the imply, median, first quartile, third quartile, minimal, and most values.The algorithm alternative accommodates eleven alternate options, comprising linear strategies (OLS, ENET), tree-based strategies (RF and GB), neural networks with one to 5 hidden layers (NN1-NN5), in addition to an ensemble of all neural networks (ENS NN) and an ensemble of all non-linear ML strategies (ENS ML). The outcomes present that the composite strategies exhibit increased imply and median portfolio returns than the opposite 9 particular person algorithms. Whereas our major focus is to not examine particular person algorithms, we discover that the neural networks (NN) show higher efficiency, whereas random forest (RF), on common, performs the worst.”
Are you searching for extra methods to examine? Join our e-newsletter or go to our Weblog or Screener.
Do you wish to study extra about Quantpedia Premium service? Examine how Quantpedia works, our mission and Premium pricing supply.
Do you wish to study extra about Quantpedia Professional service? Examine its description, watch movies, assessment reporting capabilities and go to our pricing supply.
Are you searching for historic knowledge or backtesting platforms? Examine our listing of Algo Buying and selling Reductions.
Or observe us on:
Fb Group, Fb Web page, Twitter, Linkedin, Medium or Youtube
Share onLinkedInTwitterFacebookCheck with a good friend
[ad_2]
Source link